2023/06/08
Utilization of Digital Technology in Mental Health and Psychiatry (Part 5)
The First Part: Application of Digital Technology to Major Depressive Disorder
Imaging technology, AI (Machine Learning, Deep Learning), Japan, Examination and Diagnosis, Psychiatric disorder, Patient data, Clinical Doctor, Speech technology
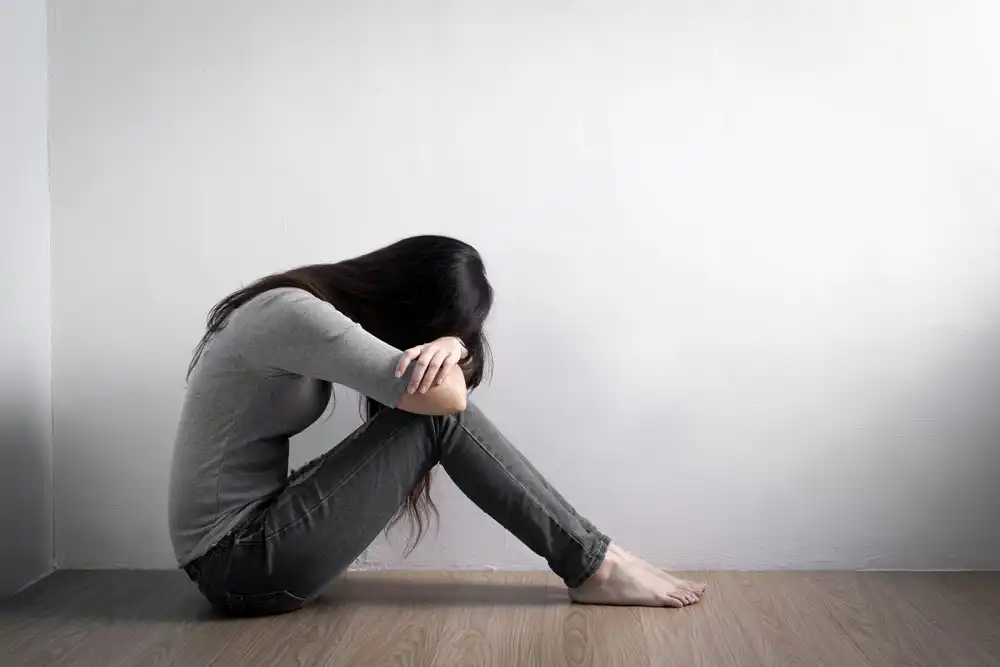
Efforts to Quantify Depressive Symptoms Using AI Technology
About Depression
Major Depressive Disorder (MDD) is a mood disorder and one of the most severe and distressing disorders affecting the psychological state of mood, motivation for activity, and interest. In Japan, the annual incidence of depression is about 1.5%, the lifetime prevalence is about 15%1), and the number of patients is increasing yearly. Depression also had a significant impact on Japan's economic losses, which were estimated to be approximately 2.7 trillion yen in a single year in 2009. 2)
Although depression presents with a wide variety of psychiatric and physical symptoms, the main symptoms include depressed mood and emotional instability, lethargy and loss of interest, sleep disturbances, and loss of appetite and concentration. These symptoms significantly impact the patient's daily life and can lead to comorbidities such as suicidal ideation and suicide attempts. It has been reported from abroad that approximately 12.6% of patients with MDD have suicidal ideation. 3)
The causes of MDD involve multiple factors, including neuroscientific in the brain, genetic, and psychological factors. MDD is also known to occur in both men and women, although the lifetime prevalence of MDD is higher in women than in men, while the rate of attempted suicide tends to be higher in men. 4)
Treatment options include medication and psychosocial treatment. However, the effectiveness of treatment varies from person to person and can sometimes be prolonged. Furthermore, the high cost of treatment can place a heavy burden on patients who engage in MDD treatment.
While there are many challenges in treating MDD, attempts to treat MDD as digital healthcare is expected to provide a new approach to these challenges and improve the quality of life of patients with MDD.
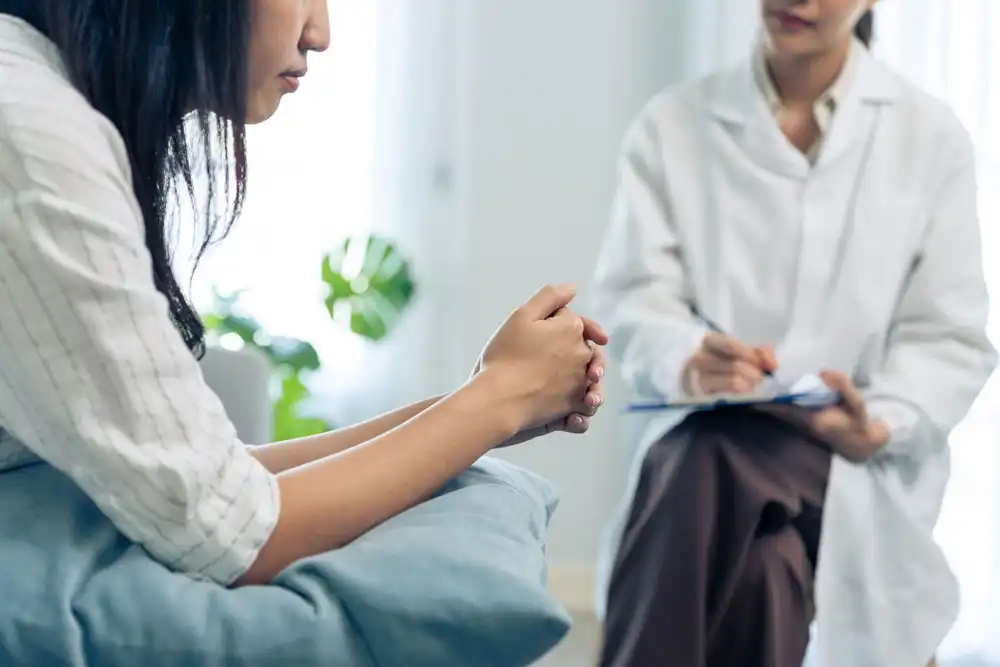
Quantification of Depressive Symptoms Using AI Technology
Diagnostic criteria for MDD exist in the International Classification of Diseases (ICD) published by the World Health Organization (WHO) and the Diagnostic and Statistical Manual of Mental Disorders (DSM) published by the American Psychiatric Association. Based on these, the actual diagnosis and evaluation of severity are made by comparing the patient with a typical patient in what respects and to what extent the patient deviates from the standard range from various perspectives.
Therefore, there is no biomarkers that can accurately and sensitively reflect the pathophysiology of the disease. The absence of biomarkers makes it difficult for non-specialists to determine the timing of treatment initiation and treatment response.
For these issues, it has been suggested that brain imaging, such as MRI, as a tool for objective diagnostic classification and prediction of treatment prognosis, may provide clues to elucidate the complex mechanisms underlying MDD. It is believed that the combined use of machine learning techniques in addition to analysis using brain imaging data may make a significant contribution to the identification of biomarkers that can differentiate MDD and predict prognosis. 5)
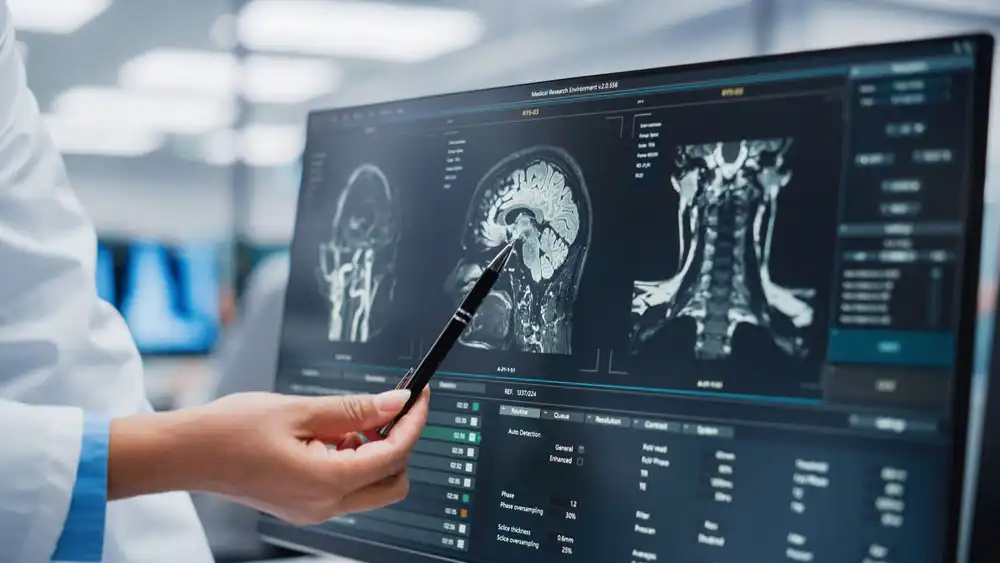
The quantification of symptoms associated with MDD has been addressed by detecting and collecting data on biological responses such as body movement, speech speed, reaction time, speaking style, sleep, and skin temperature.
For example, there is a report on the evaluation of depression using a combination of data obtained from automatic speech analysis and transition learning. The report showed that it might be possible to distinguish whether a person is in a remission phase of depression or not by comparison with a healthy person if the noise was removed. 6) Not only is it non-invasive, but it is also time cost-effective as it is possible to evaluate even with short periods of speech, which is an advantage and may be a promising biomarker.
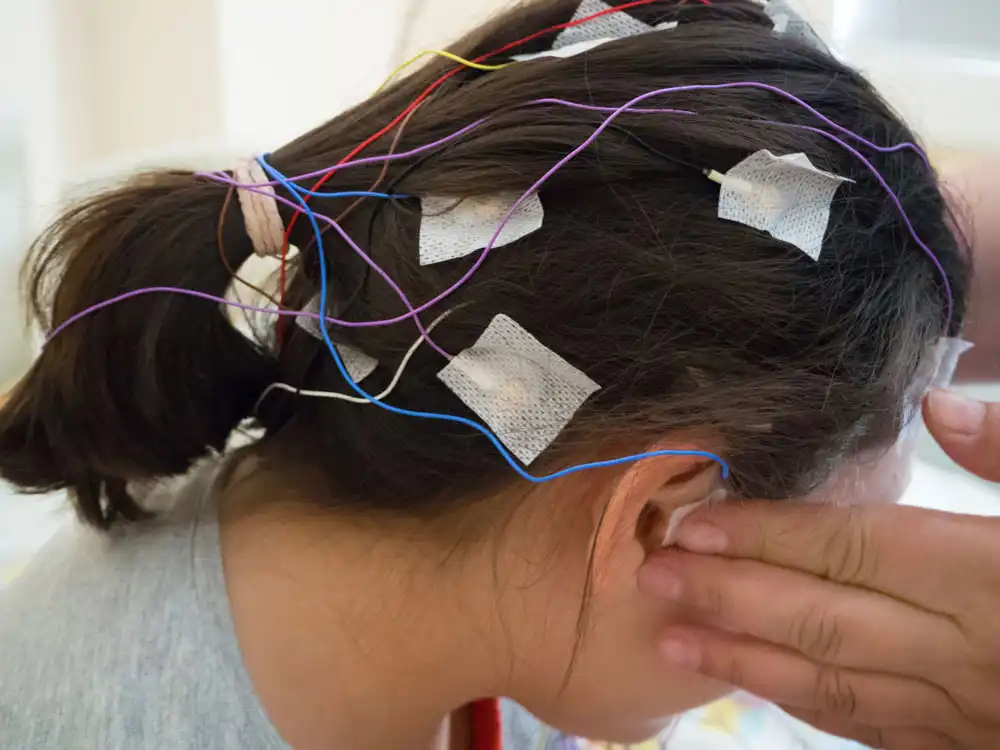
Another study reported that examined whether a multimodal machine learning model could work effectively in differentiating depression from normal subjects using three different modalities: electroencephalography of the frontal lobe, eye tracking, and a galvanic skin response (electrical conductivity of the skin) sensor. The results suggest combining the three modalities is generally more accurate and reproducible. 7) However, limitations include the relatively high cost of measurement using each modality and the need for professional assistance to perform the measurement. Therefore, how to solve the problem of accessibility will require further discussion in the future.
(To be continued to the next issue)
【Source】
Table 1-26 Number of deaths and death rates (per 100,000 population) by sex and causes
(the condensed list of causes of death for Japan) (Excel:23KB),
https://www.mhlw.go.jp/english/database/db-hh/1-2.html(Accessed 2023-06-07)
We have not received any rewards from the publishers for writing this manuscript.
The information contained in this article is compiled by the respective authors based on publicly available information. We assume no responsibility whatsoever for any damage or disadvantage caused by actions taken based on such information. Unauthorized reproduction of articles, photos, charts, etc. is prohibited.
Copyright © 2023 LSMIP / CM Plus Corporation
A Series of Articles